9.3. Linear Systems and Regression#
using LinearAlgebra
9.3.1. Linear Systems#
One of the most common uses of matrices is for solving linear systems of equations. Julia uses the backslash operator \
for this:
A = [1 2; 3 4]
b = [5,1]
x = A \ b # Solve Ax = b for x
A*x == b # Confirm solution is correct
true
One way to view the syntax A\b
is that it multiplies by A
-inverse from the left, but using much more efficient and accurate algorithms.
For systems with many right-hand side vectors b
, the \
operator also works with matrices:
B = [5 7; 1 -3]
X = A \ B # Solve for two RHS vectors
A*X == B
false
The algorithm used by the \
operator is typically Gaussian elimination, but the details are quite complex depending on the type of matrices involved. Due to the high cost of general Gaussian elimination, it can make a big difference if you use a specialized matrix type:
n = 2000
T = SymTridiagonal(2ones(n), -ones(n)) # n-by-n symmetric tridiagonal
for rep = 1:3 @time T \ randn(n) end # Very fast since T is a SymTridiagonal
Tfull = Matrix(T) # Convert T to a full 2D array
for rep = 1:3 @time Tfull \ randn(n) end # Now \ is magnitudes slower
0.186211 seconds (380.23 k allocations: 23.836 MiB, 99.95% compilation time)
0.000041 seconds (4 allocations: 63.000 KiB)
0.000048 seconds (4 allocations: 63.000 KiB)
0.300326 seconds (297.61 k allocations: 50.643 MiB, 3.03% gc time, 59.62% compilation time)
0.127201 seconds (5 allocations: 30.564 MiB, 5.90% gc time)
0.120655 seconds (5 allocations: 30.564 MiB)
The matrix A
in A\b
can also be rectangular, in which case a minimum-norm least squares solution is computed.
9.3.2. Linear regression#
Suppose you want to approximate a set of \(n\) points \((x_i,y_i)\), \(i=1,\ldots,n\), by a straight line. The least squares approximation \(y=a + bx\) is given by the least-squares solution of the following over-determined system:
x = 0:0.1:10
n = length(x)
y = 3x .- 2 + randn(n) # Example data: straight line with noise
A = [ones(n) x] # LHS
ab = A \ y # Least-squares solution
using PyPlot
xplot = 0:10;
yplot = @. ab[1] + ab[2] * xplot
plot(x,y,".")
plot(xplot, yplot, "r");
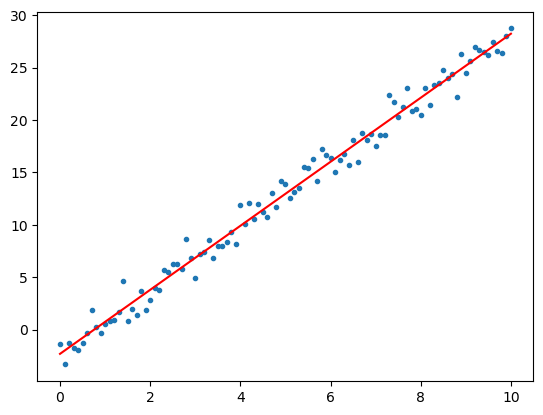